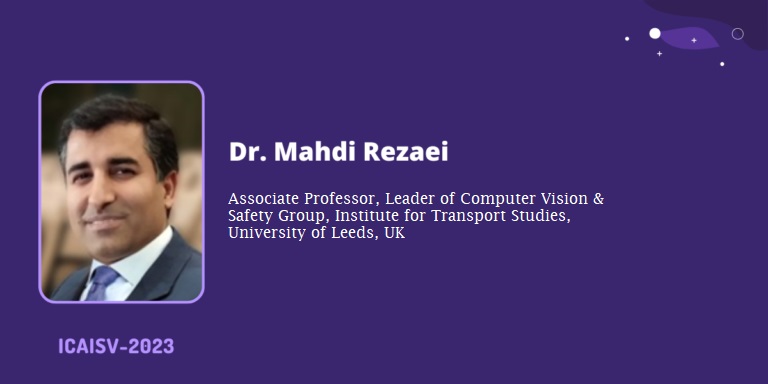
Local and Global Contextual Features Fusion for Pedestrian Intention Prediction
Biography: Dr Mahdi Rezaei is an Associate Professor of Computer Science and a University Academic Fellow at the Institute for Transport Studies, University of Leeds. Dr Rezaei has a background in AI, Computer Vision, and Machine Learning and obtained his PhD from the University of Auckland, New Zealand. Having over 15 years of experience in academia and industry his primary research focus is the application of Computer Vision and ML in Autonomous Vehicles, Smart Cars, and Driver/Occupant Behaviour Monitoring. He is a member of the Academic Advisory Group in the LIDA data scientist development programme (LIPAG), and also an executive member of the “Universities Transport Study Group” (UTSG). Dr Rezaei has contributed as a PI or lead discipline CoI in various European and UK-funded projects, totalling over £3 million.
Abstract: Autonomous vehicles (AVs) are becoming an indispensable part of future transportation. However, safety challenges and lack of reliability limit their real-world deployment. Towards boosting the appearance of AVs on the roads, the interaction of AVs with pedestrians including “prediction of the pedestrian crossing intention” deserves extensive research.
This is a highly challenging task as involves multiple non-linear parameters.
In this direction, we extract and analyse spatio-temporal visual features of
both pedestrian and traffic contexts. The pedestrian features include body pose and local context features that represent the pedestrian’s behaviour.
Additionally, to understand the global context, we utilise location, motion,
and environmental information using scene parsing technology that represents the pedestrian’s surroundings, and may affect the pedestrian’s intention. Finally, these multi-modality features are intelligently fused for effective intention prediction learning. The experimental results of the proposed model on the JAAD dataset show a superior result on the combined AUC and F1-score compared to the state-of-the-art.