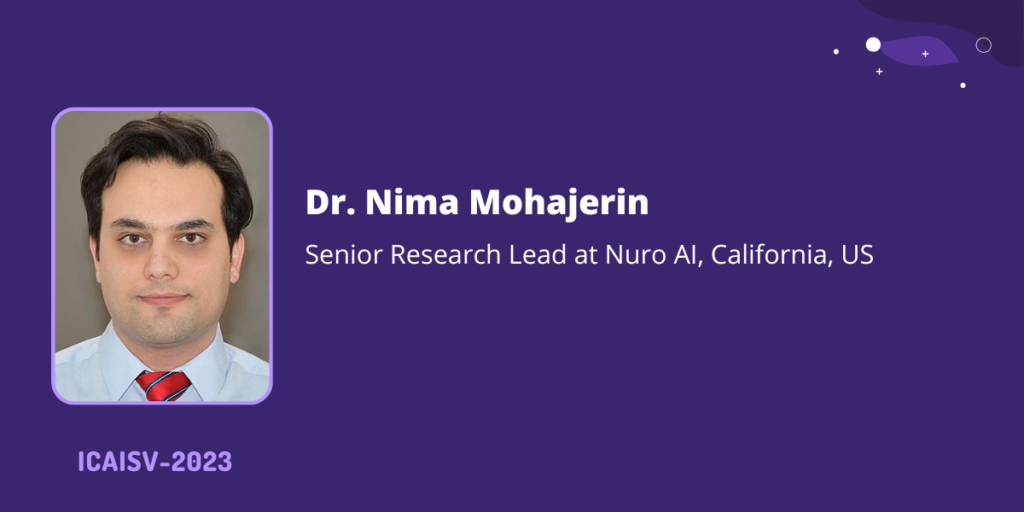
Long-Tail Learning and Out-of-Distribution Detection in Autonomous Driving
Biography:
Nima Mohajerin is an accomplished researcher and engineer with a keen interest in the intersection of Machine Learning and Robotics. He has been an active participant in the Autonomous Vehicle industry and academia since 2012, where he has made significant contributions to the research and development of cutting-edge technologies. Currently, he serves as a Senior Research Lead at Nuro AI, a position he assumed after serving as a Senior ML researcher in Microsoft Applied Science Group (ASG). Nima obtained his B.Sc in Electrical Engineering from Shahid Beheshti University in 1998, and his M.Sc in Control from Amirkabir University in 2003. After several years of experience in the automobile industry in Iran, he moved to Sweden and joined AASS labs in Orebro Sweden, where he obtained another M.Sc in Computer Science and Robotic Intelligent Systems in 2012. Nima then relocated to Canada, where he obtained his Ph.D. in the Mechatronics and Mechanical Engineering department of the University of Waterloo in 2017.Throughout his career, Nima has gained considerable expertise in various ML methods, including RNNs, Bayesian Inference, Generative models, Reinforcement Learning, Fuzzy Logic Controllers, Density Estimation, among others. His primary research interests revolve around the application of these techniques to solve complex problems in the Robotics field.
Abstract: Supervised Learning (SL) is predominantly the most often employed method in most of Machine Learning approaches in industry. One reason, perhaps, is the fact that collecting and labeling more data is always available and high-end processing power is more accessible. However, in applications where the ML algorithm must interact with the physical world, there is another important factor in play; that is, the rareness of objects and events. Examples include: unexpected objects on road (e.g., a tree branch), accidents scenes, parades, construction sites, etc. However, a rare event or object usually requires a dramatic change in the expected behaviour of the ego vehicle, hence, should be peroperly detected by the onboard system. The main challenges in detecting rare objects/events are the imbalance in the training dataset and, by nature, the limited amount of information that is carried by the training set. In this talk, the problem of Long-Tail Learning and Out-of-Distribution detection is introduced and state-of-the-art will be briefly summarized.